Enhancing your reserving process with effective validation
Insurance consulting Reserving Capital modelling and validation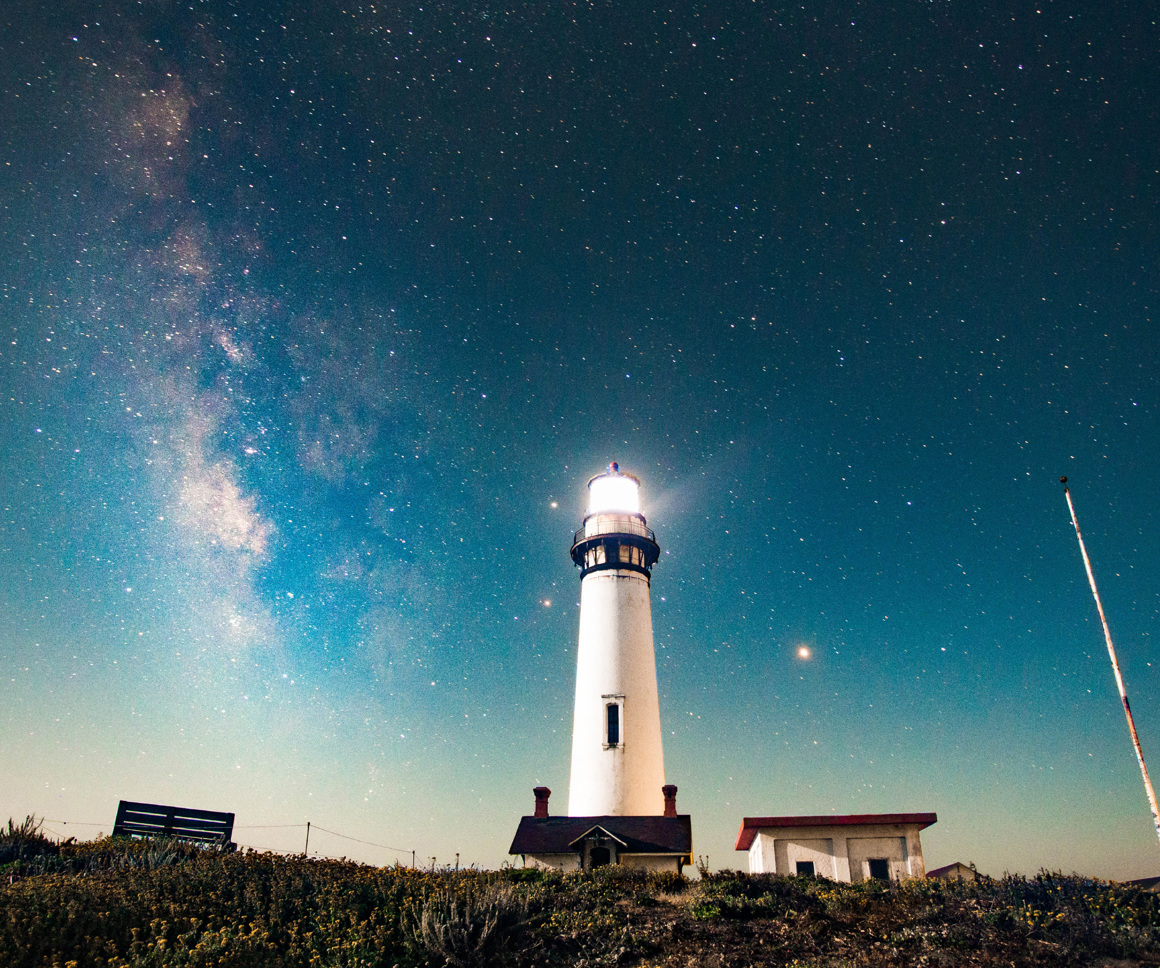
Reserving teams are a vital artery of feedback on business performance, with stakeholders demanding faster turnarounds and ever greater insights into reserves, loss ratios, and performance trends.
Reserving validation is all about making sure that those reserving results are reasonable best estimates and that emerging trends (that could invalidate key assumptions) are identified at the earliest possible stage.
This article explores some of the pitfalls of existing approaches to reserving validation, how to assemble an effective overall framework, and how to enhance that framework using machine learning techniques.
Validation pitfalls
Typically there are three key areas where reserving validation processes fall short of their potential.
First, these techniques are often applied in a scattergun manner without an overall framework or plan. This risks coverage gaps and a false sense of security.
Second, validation efforts tend to focus on past (known!) problem areas. The most heavily validated reserving classes tend to be those which have experienced recent deteriorations, rather than those perhaps most at risk of future problems.
Third, unless well designed, validation processes can be inefficient compared to their perceived value add. Ensuring comprehensive coverage of reserving classes and metrics can be time-consuming and feel unrewarding. After all, most classes will pass validation testing most of the time.
In the remainder of this article, we consider how to address these pitfalls and design an effective validation process.
Build your framework “top down”
Designing the validation framework from the top down helps eliminate coverage gaps, which makes the overall validation process more robust.
To design a top-down framework, a one-off exercise is needed to:
- Map out the potential drivers of adverse movement across the portfolio, considering a wide range of historical examples, market issues, and scenarios.
- Back-test and scenario-test a range of diagnostics using historical examples of reserve deteriorations and trends. Use this testing to help select diagnostics for your validation framework that are most responsive to the types of trends and deteriorations you regularly see.
- Set quantitative validation tolerances, based on the back-testing and scenario-testing, to automatically flag classes or metrics that are exhibiting abnormal behaviour.
Although the validation framework may be new, the techniques that sit within it probably won’t be. Many tried and tested reserving tools are useful in a validation context (eg actual versus expected analysis and various KPI graphs). The added value comes from bringing multiple techniques together to compensate for the limitations of any individual approach.
Let machine learning do the heavy lifting
Using machine learning allows a validation process to cover more diagnostics for more classes in less time. This makes the overall validation process more effective without becoming excessively time consuming.
In this context, machine learning can be used to perform diagnostics on ultimate projections and underlying assumptions. These diagnostics can range from general statistical tools, such as goodness of fit of assumptions to data, through to reserving specific trend detection features, such as inflationary pressures, step changes in performance, or isolated accident quarters that are behaving unexpectedly.
Reserving validation is first and foremost a coverage challenge. A validation process that runs a wider range tests and diagnostics on a large number of classes is more likely to identify reserving risks, trends, and useful business feedback than a more restricted process.
In the past, the breadth of validation has been limited by practical constraints – ie the sheer number of diagnostics that have to be manually reviewed. By automating the process, you can use machine learning to automatically flag poorly behaving diagnostics for review. This helps immediately focus the reviewing actuary’s time more on the challenging areas, without them needing to spend time on classes where models are already well fitted.
Machine learning approaches needn’t be complex to deploy. Open-source packages in “R” and Python increasingly offer pre-built functions that can help with simple automated diagnostics. Commercial packages which are more tailored to the insurance industry, like LCP InsurSight, are also available.
Use visualisations to guide your decision making
As machine learning allows the validation process to grow and accommodate more segmentations and diagnostic tests, good visualisation of diagnostics becomes ever more important.
Visualisations of key metrics help the reserving team quickly understand the issues being flagged by the machine learning tool. This in turn helps the team to ensure that each issue is appropriately allowed for in the underlying reserving assumptions, and also to clearly articulate each trend / risk to the reserving committee.
Extending validation to different granularities
The combined efficiency achieved with machine learning and quality data visualisation means that validation of reserves can increasingly be extended in ways that previously weren’t practical.
An example of this is to validate reserves by considering more granular segmentations than the standard reserving granularity.
Granular validation can be very powerful because the assumption that claims in each reserving segment develop homogenously is both critical and often untested. Allocating reserves within each segment (eg motor own-damage) down to more granular sub-segments (eg vehicle type, or front vs side or rear impact) and then validating those sub-segments can help identify instances where there is heterogeneity within a reserving class.
Identifying heterogeneity often helps explain the driver of otherwise poorly understood trends at the reserving granularity, as well as indicating alternative reserving granularities that may be more homogenous for future modelling.
The future for reserving validation
By combining a top-down framework, intelligent use of machine learning, high-impact visual diagnostics, and an expansion of validation activity to different granularities, the overall validation process becomes much more effective.
A reserving function with an effective validation process will set ultimates that that are likely more reliable and less prone to deterioration (or improvement!). Effective validation also allows for better identification of reserving trends at multiple granularities.
Collectively, the benefits of effective reserving validation strengthen the virtuous cycle of reserving feedback into business planning, giving firms a competitive advantage.
As published in The Actuarial Post, April 2022. Original article here.